Blog
Innovating Industry Insights
Stay informed with our latest blog articles.
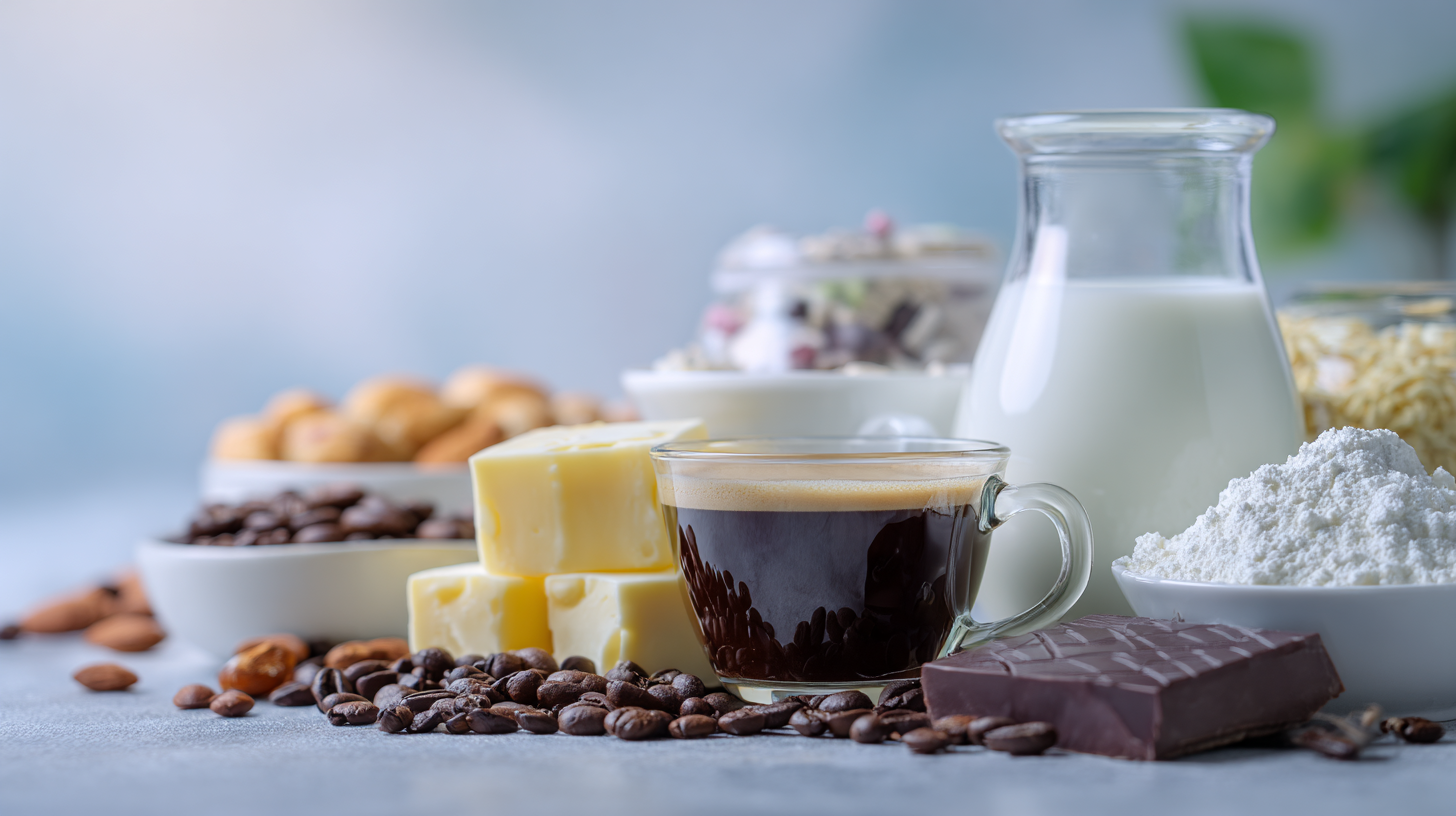
Latest post
Maximizing Performance in Food & Beverages Manufacturing with TrendMiner
July 14, 2025
From coffee beans to chocolate bars, leading food-and-beverage plants are supercharging throughput and slashing downtime with TrendMiner’s real-time analytics. Discover how data-driven insights turn cleaning cycles, evaporator fouling, and pasteurization hiccups into opportunities for 24/7 efficiency and consistent, top-quality products.
Filters
Thank you! Your submission has been received!
Oops! Something went wrong while submitting the form.