Blog
Innovating Industry Insights
Stay informed with our latest blog articles.
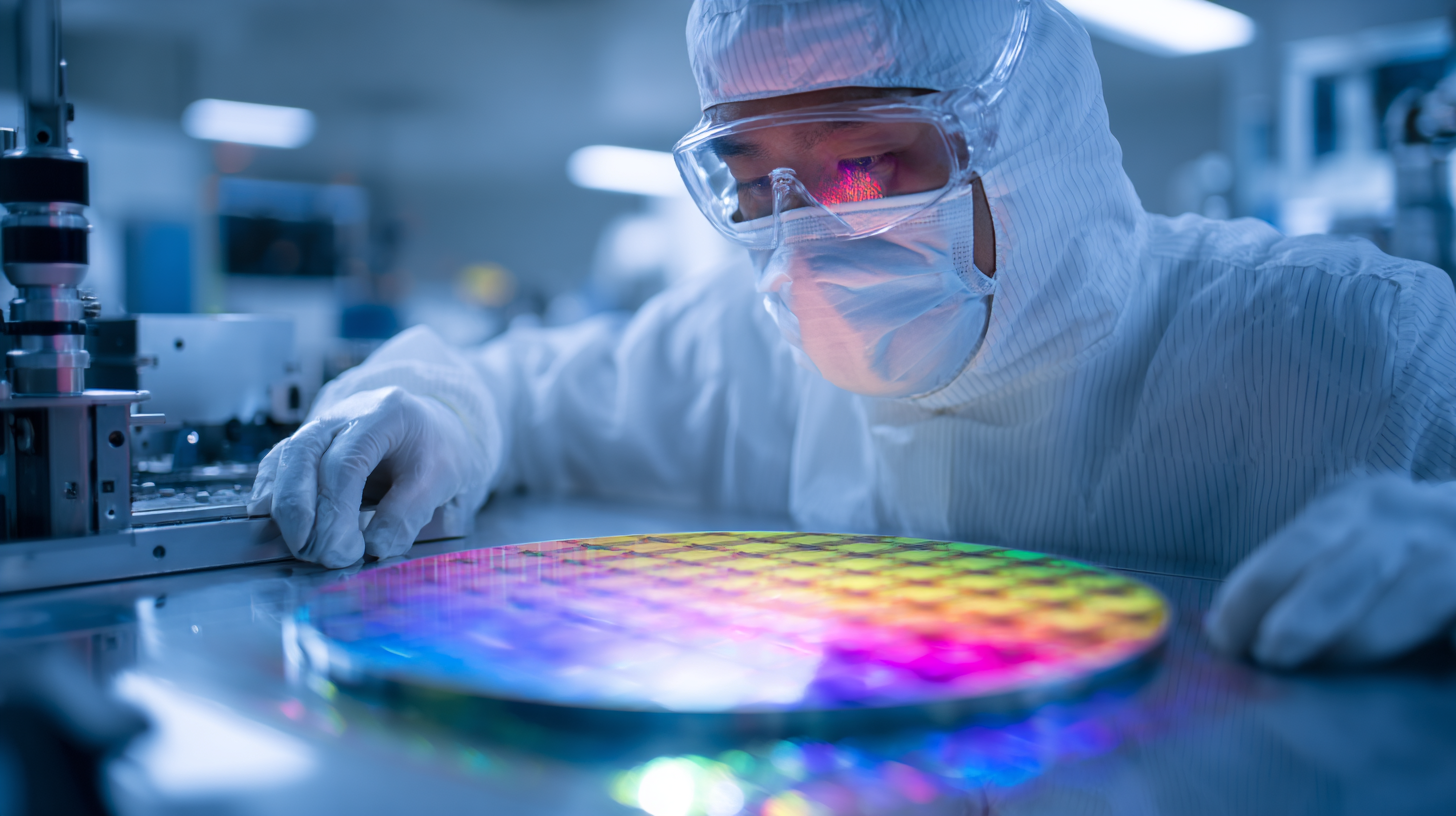
Latest post
Optimize Cleanroom Performance & Energy Use
July 10, 2025
TrendMiner’s self-service industrial analytics streamlines cleanroom monitoring: instantly visualize time-series data, detect inefficiencies in ultra-pure air systems, and cut energy costs while protecting GMP and ISO-compliant conditions — no coding required.
Filters
Thank you! Your submission has been received!
Oops! Something went wrong while submitting the form.